Neural Bokeh: Learning Lens Blur for Computational Videography and Out-of-Focus Mixed Reality
Conference paper at IEEE VR 2024
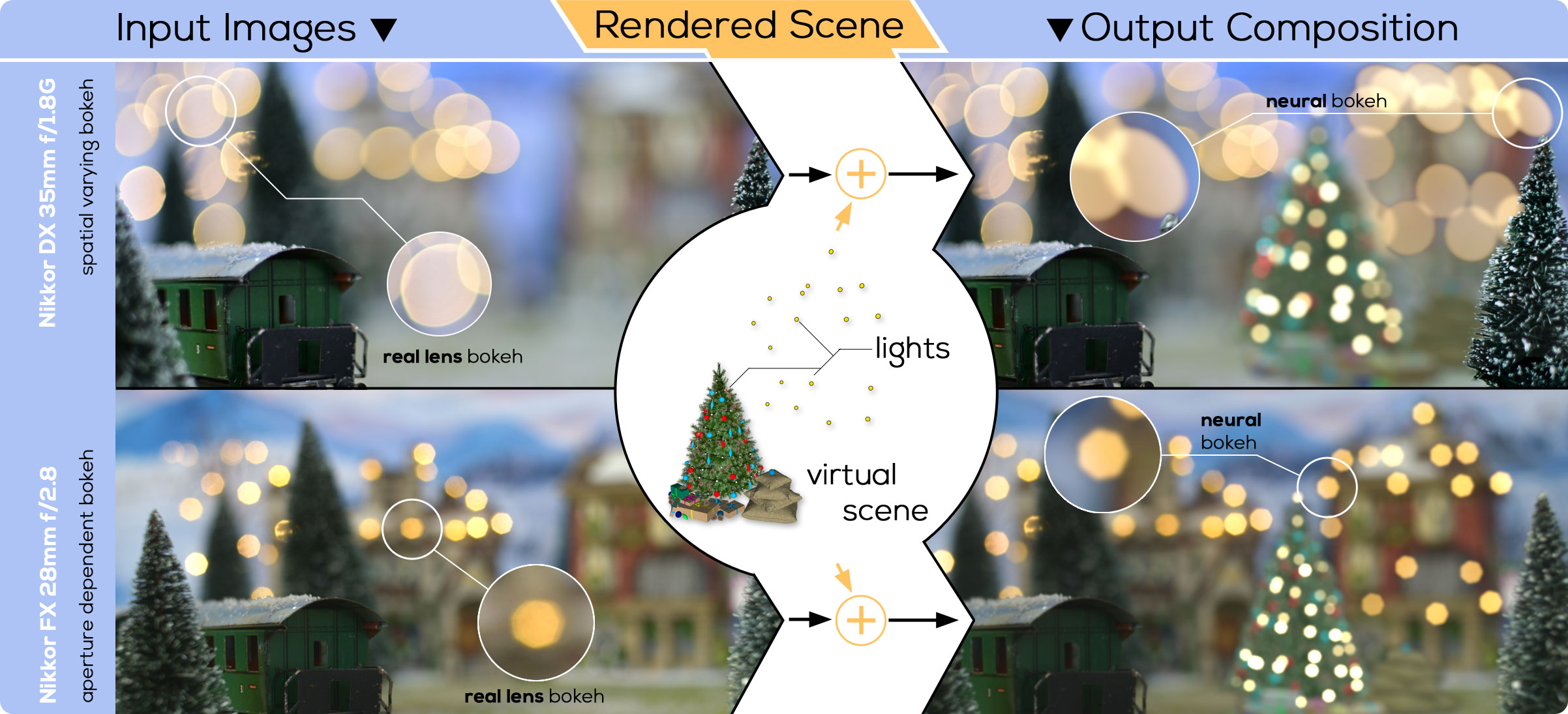
Abstract
We present Neural Bokeh, a deep learning approach for synthesizing convincing out-of-focus effects with applications in Mixed Reality (MR) image and video compositing. Unlike existing approaches that solely learn the amount of blur for out-of-focus areas, our approach captures the overall characteristic of the bokeh to enable the seamless integration of rendered scene content into real image, ensuring a consistent lens blur over the resulting MR composition. Our method learns spatially varying blur shapes, from a dataset of real images acquired using the physical camera that is used to capture the photograph or video of the MR composition. Accordingly, those learned blur shapes mimic the characteristics of the physical lens. As the run-time and the resulting quality of Neural Bokeh increase with the resolution of input images, we employ low-resolution images for the MR view finding at runtime and high-resolution renderings for compositing with high-resolution photographs or videos in an offline process. We envision a variety of applications, including visual enhancement of image and video compositing containing creative utilization of out-of-focus effects.
Video
Example Results
Select camera
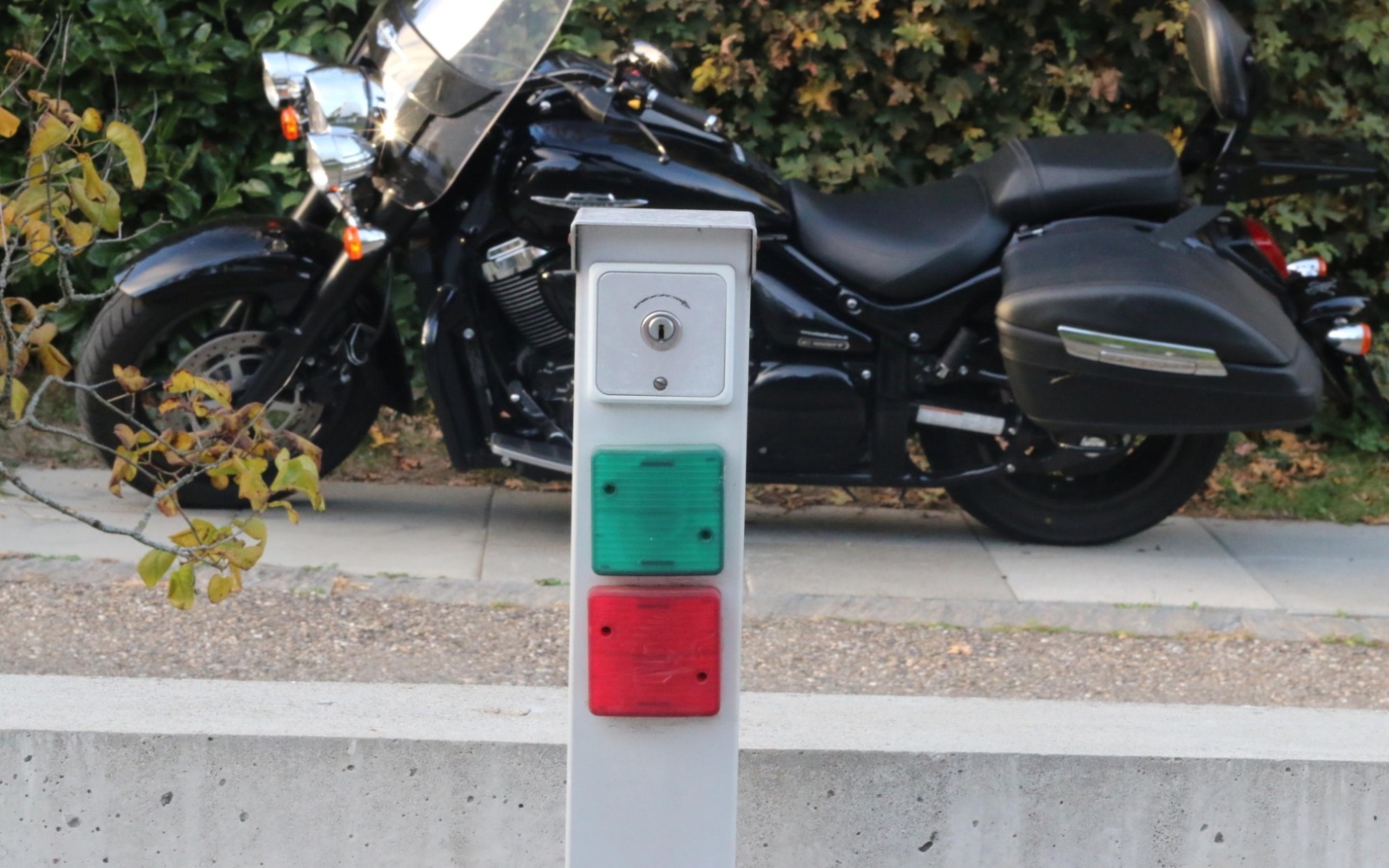
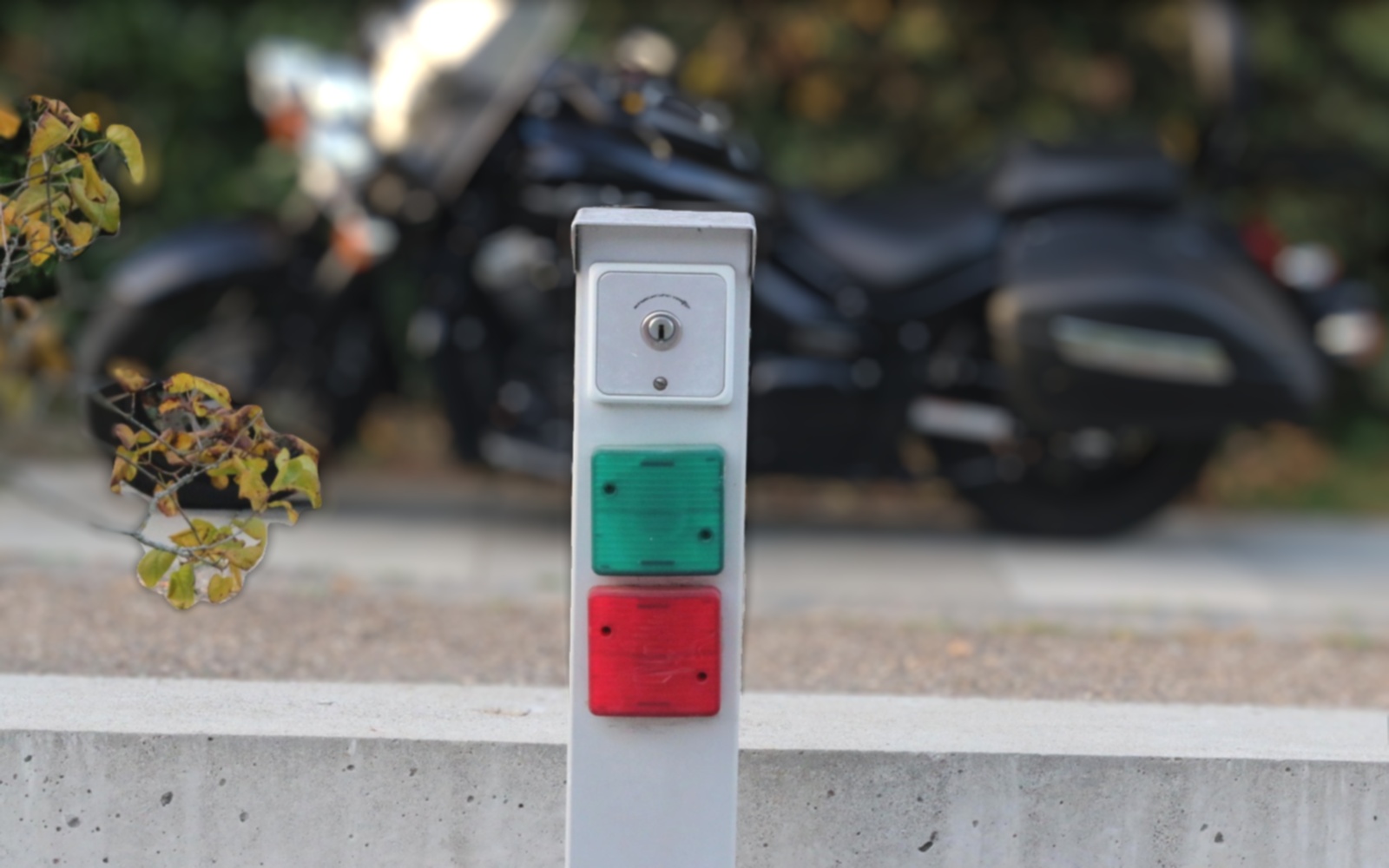
Select camera
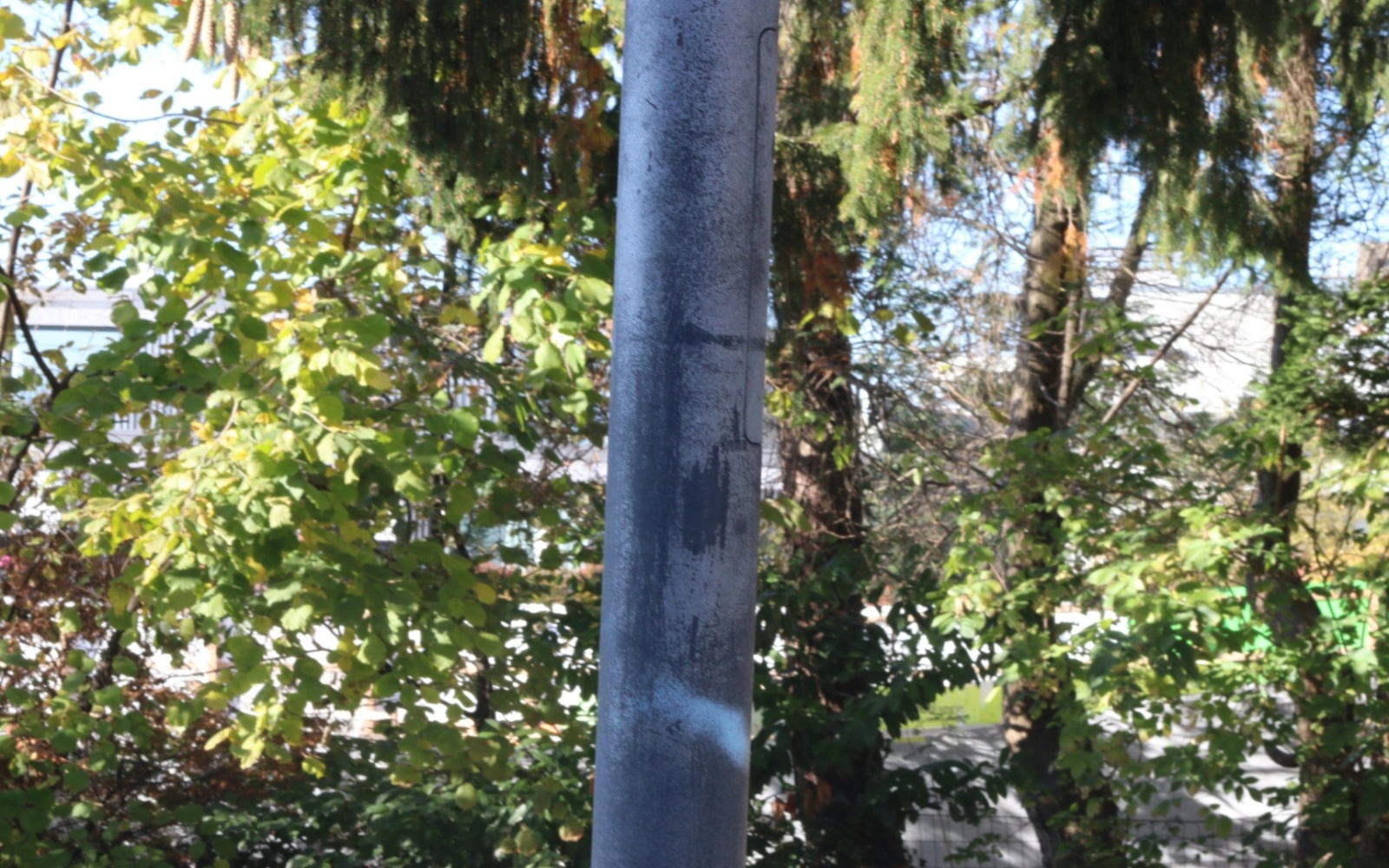
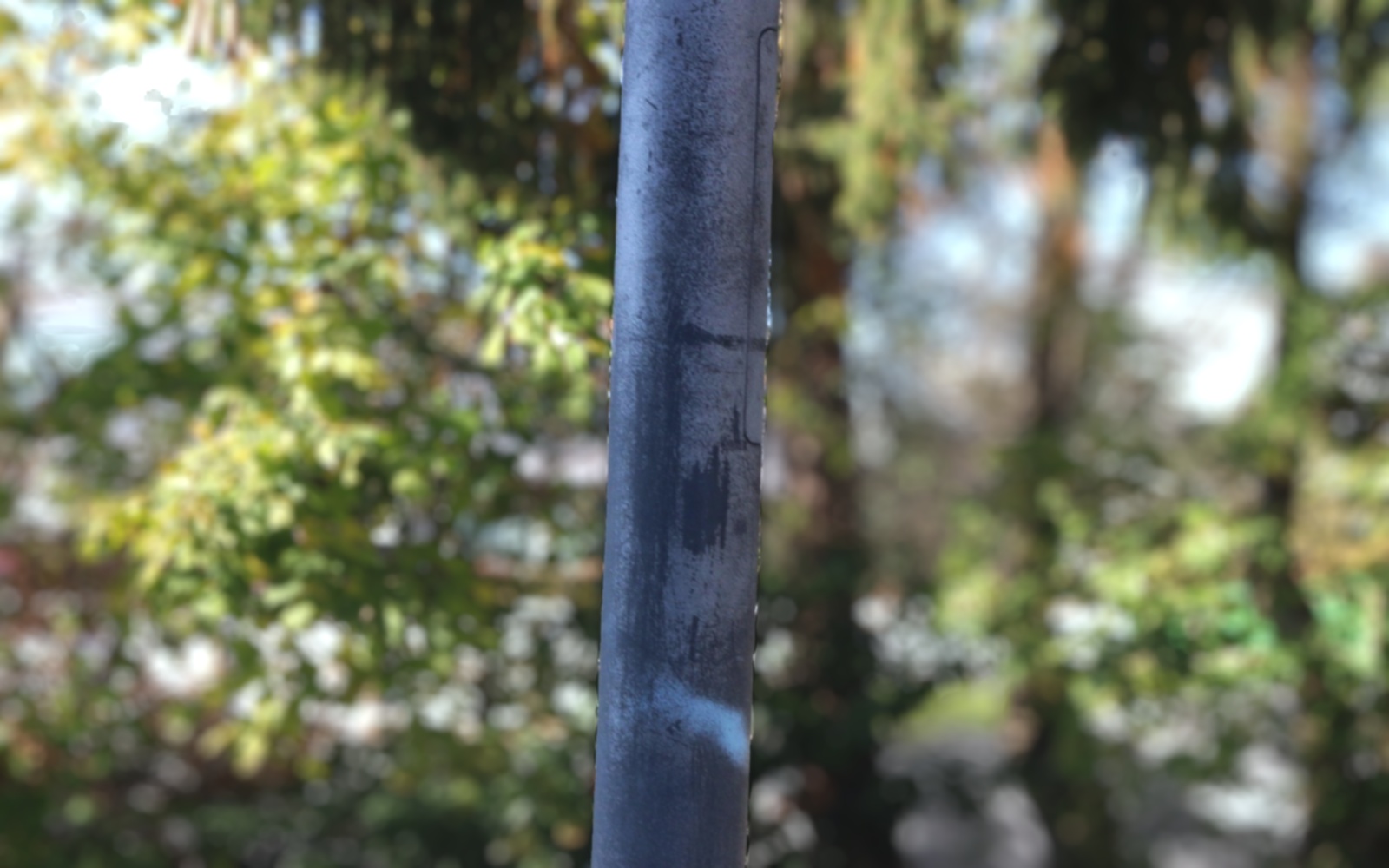
Select camera
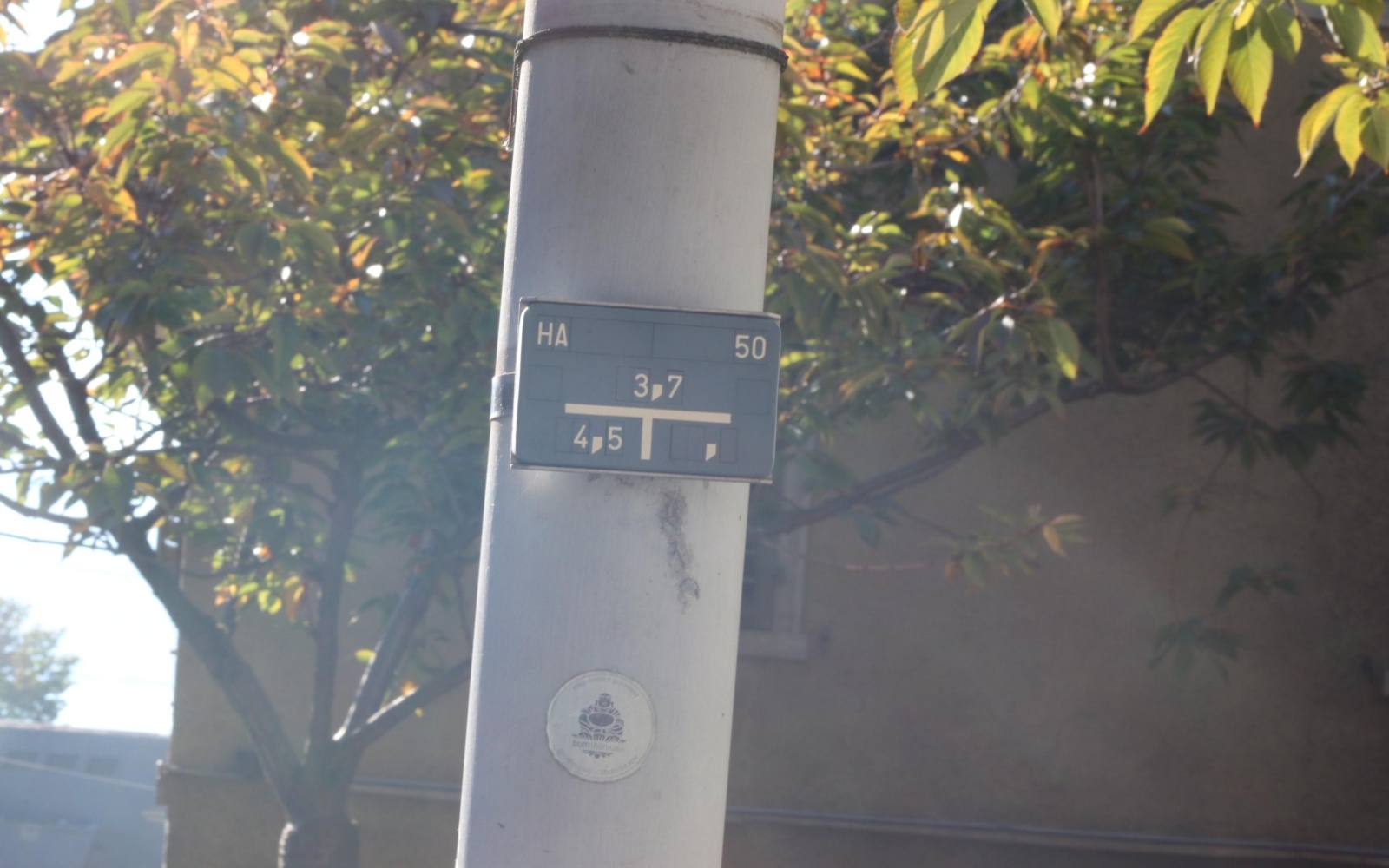
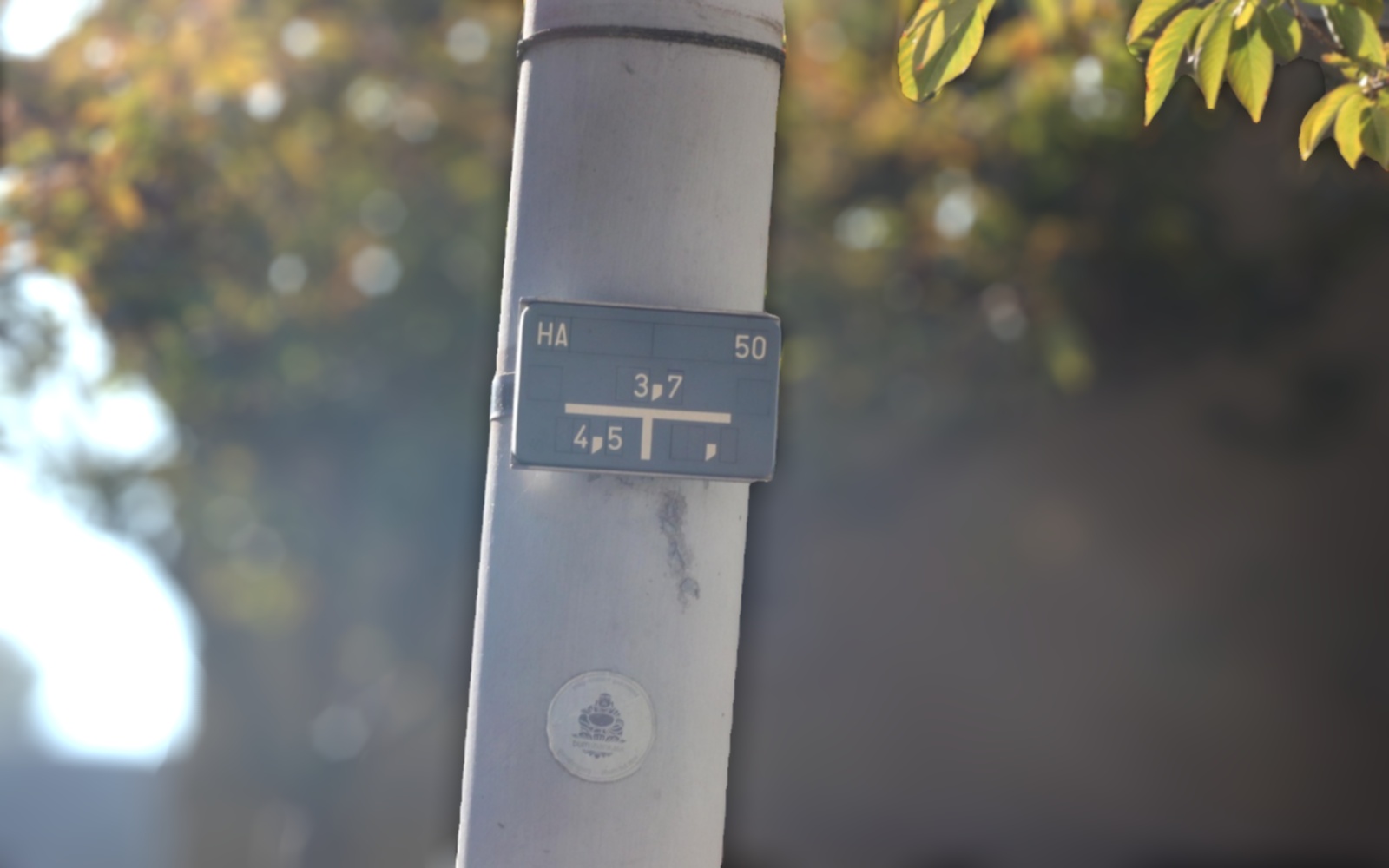
Select camera
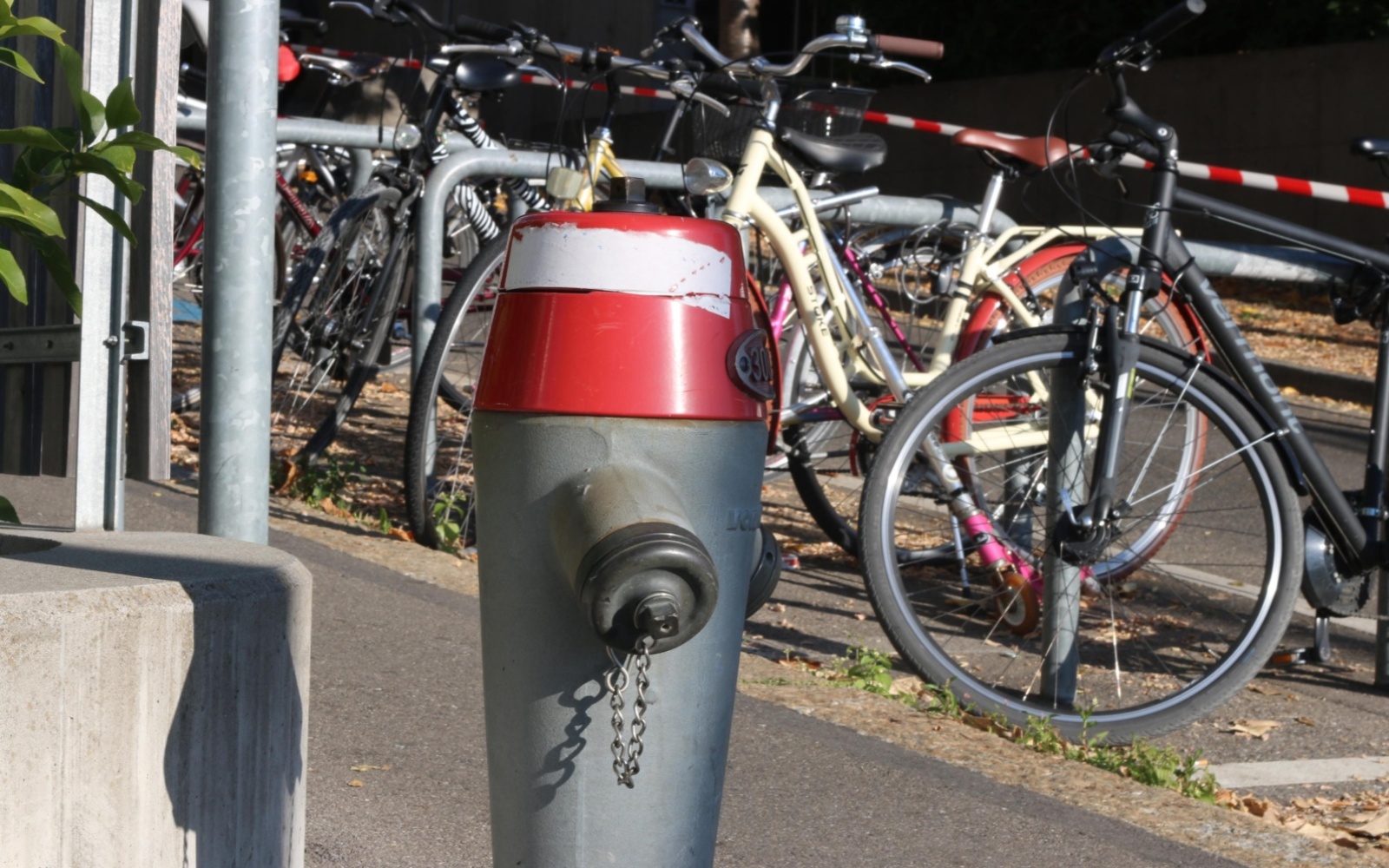
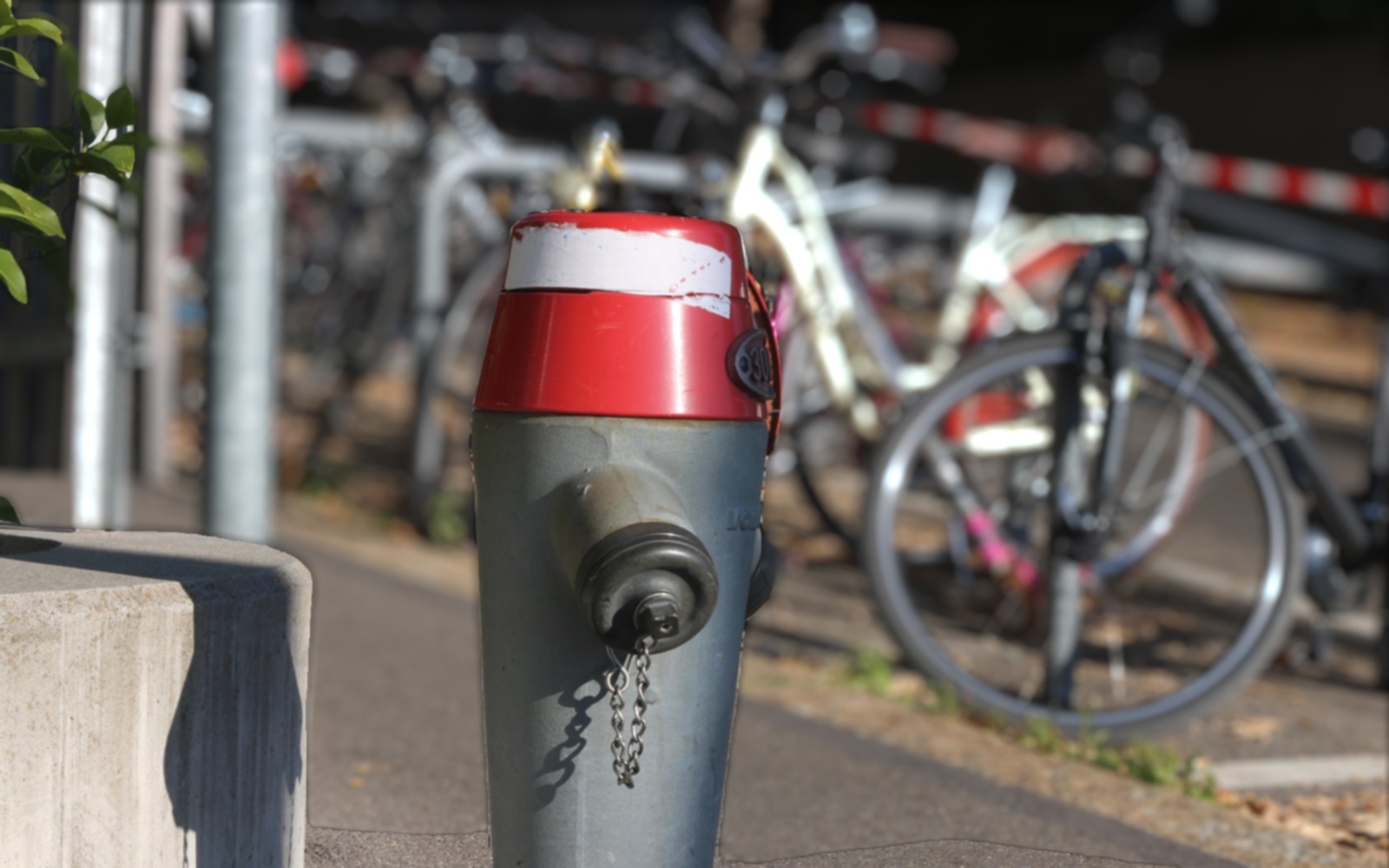
Select camera
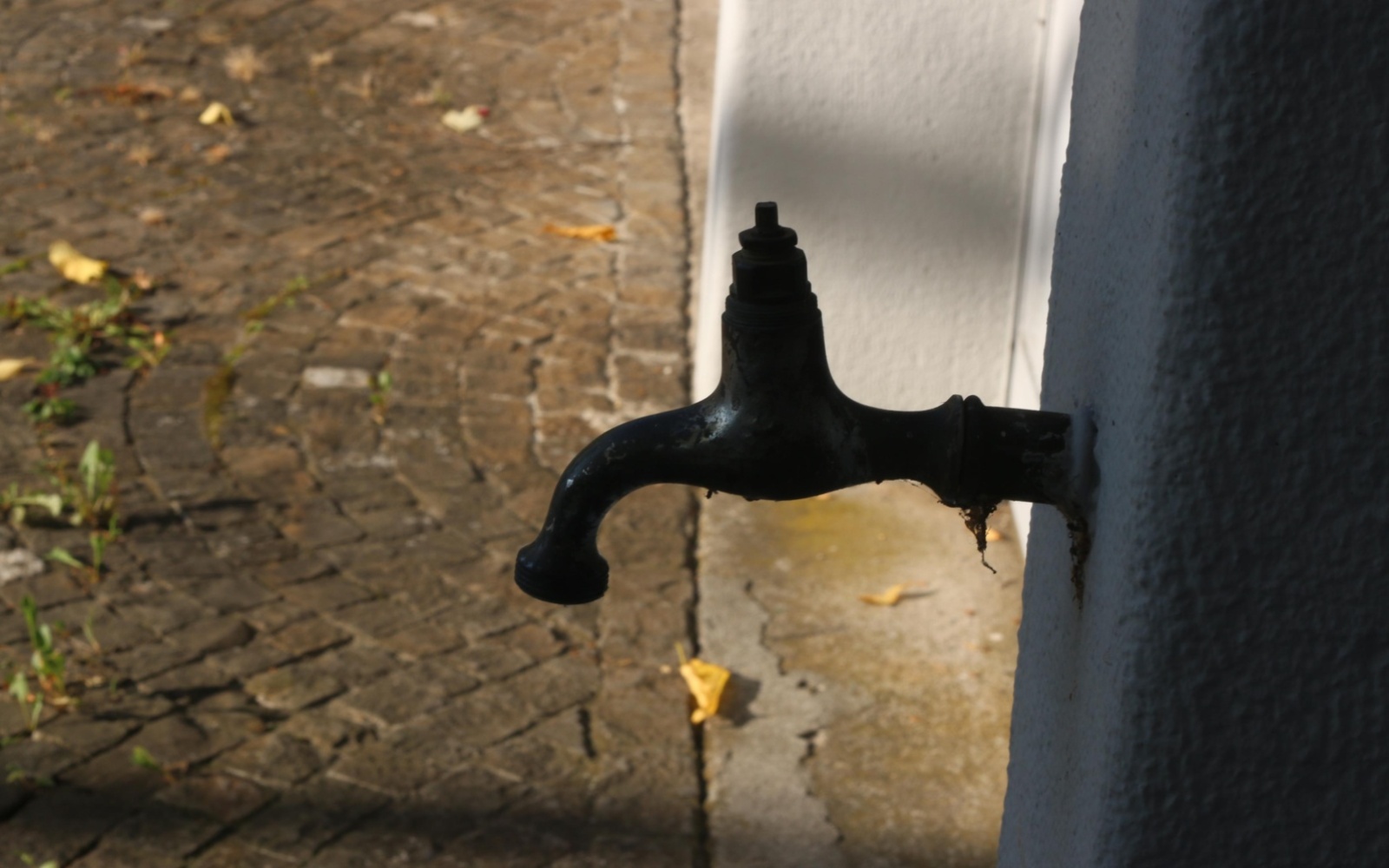
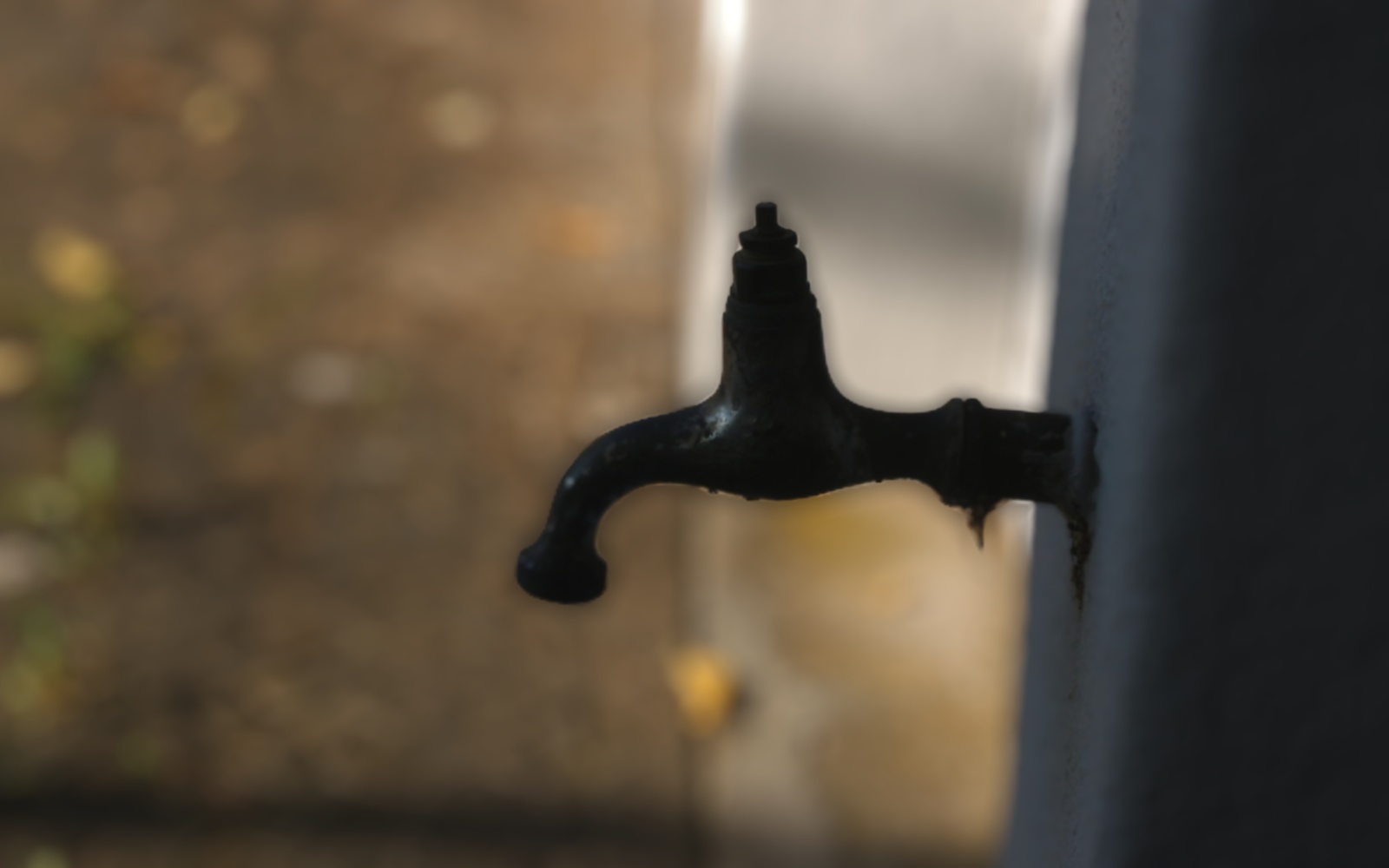
Select camera
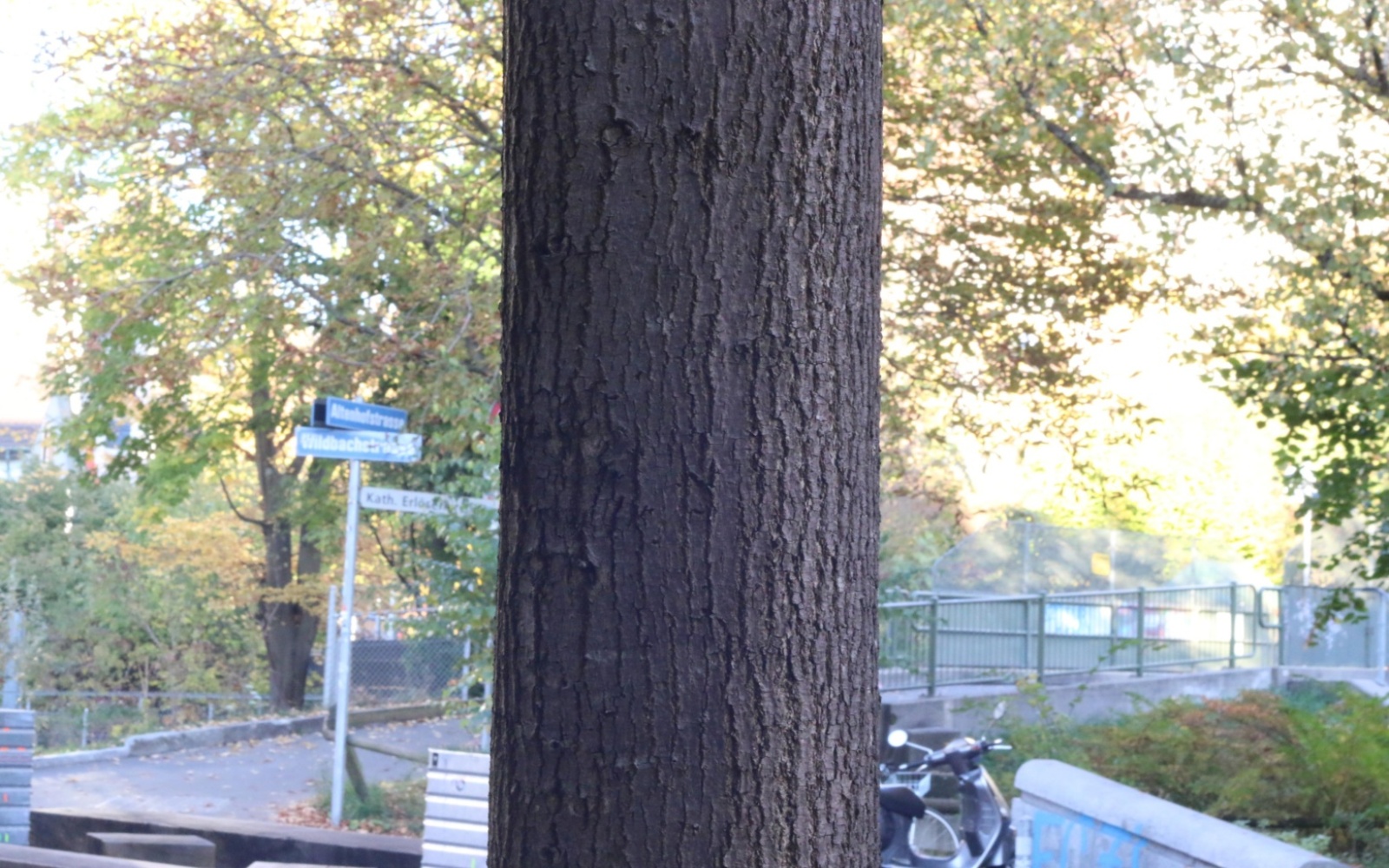
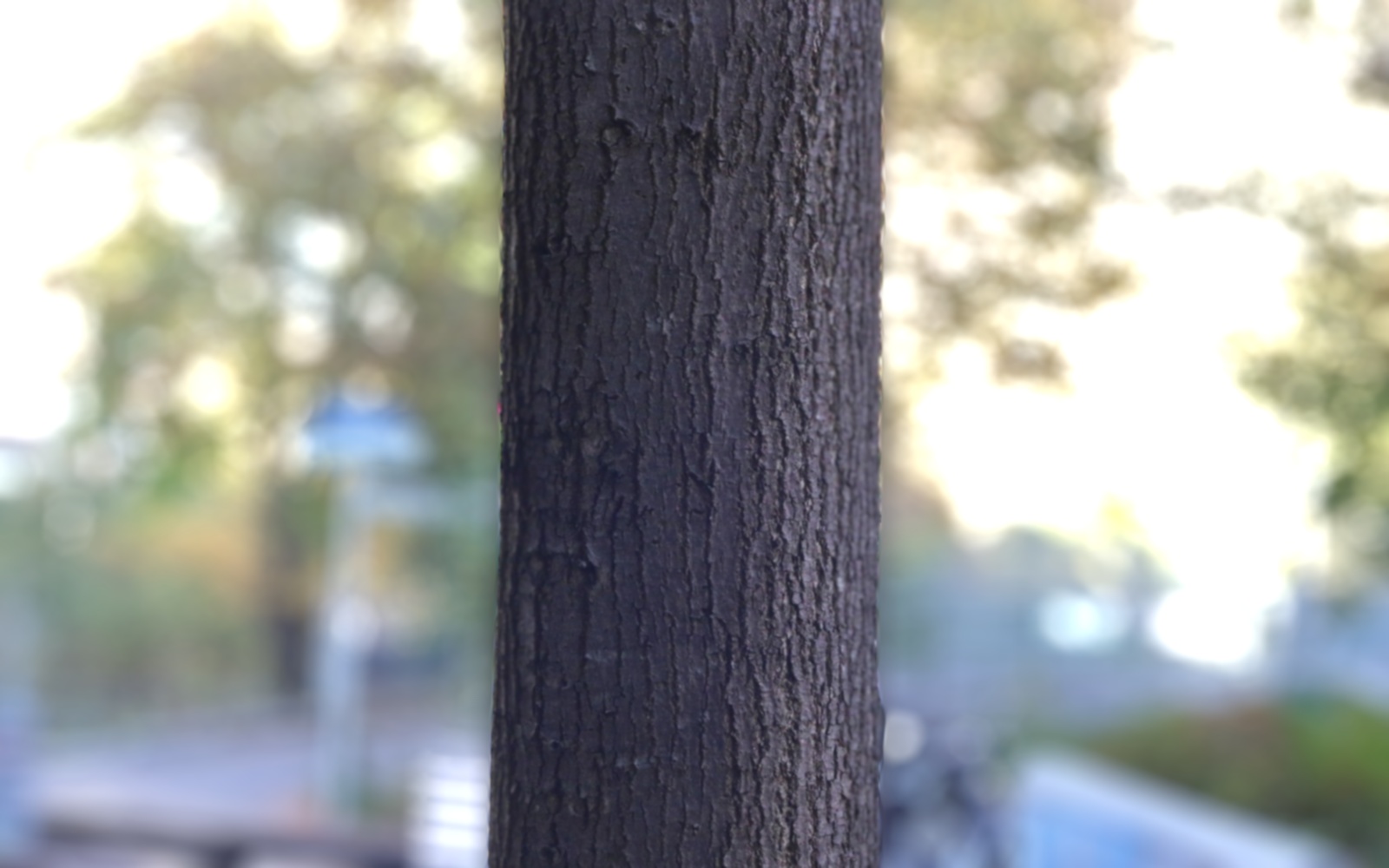
Select camera
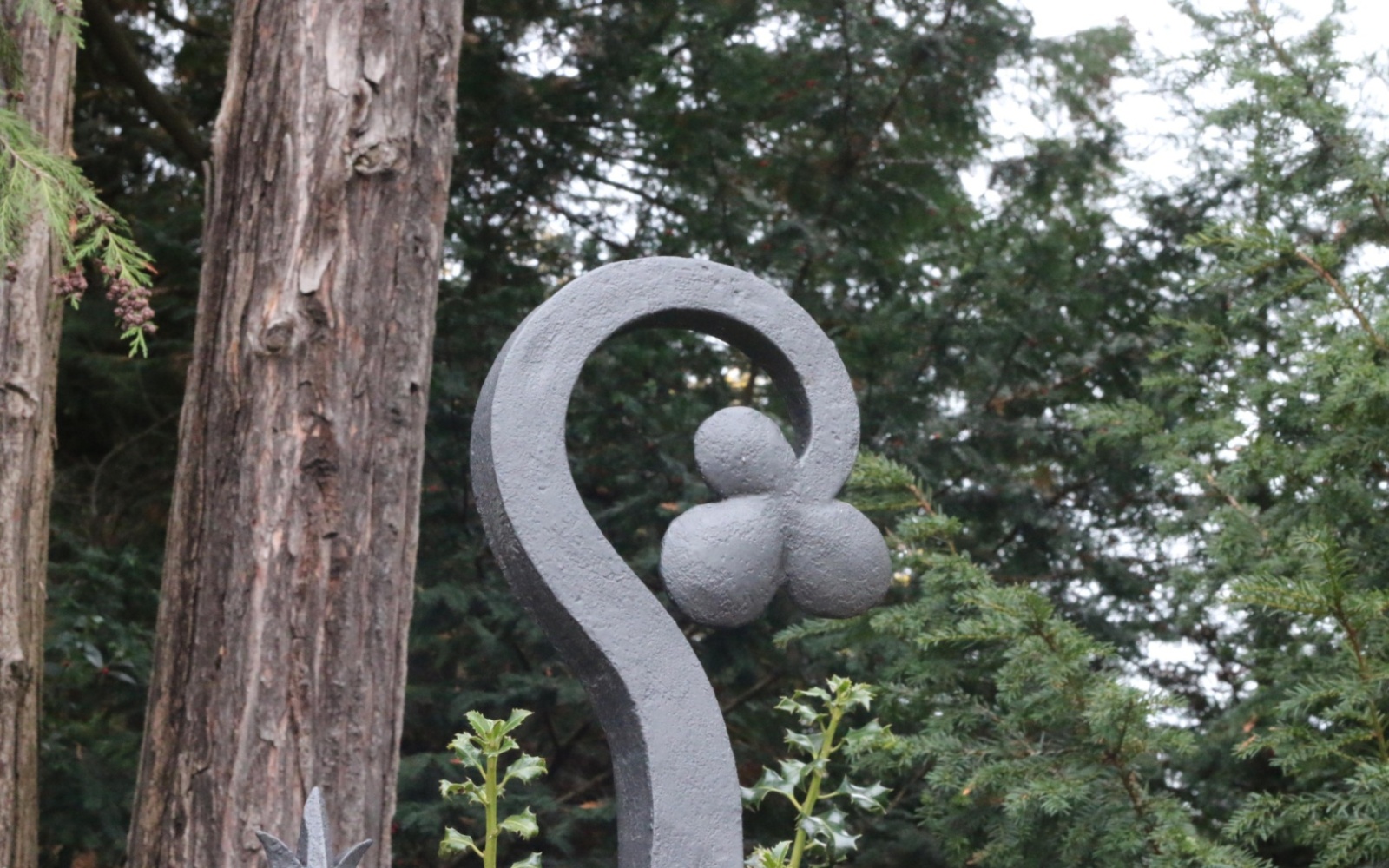
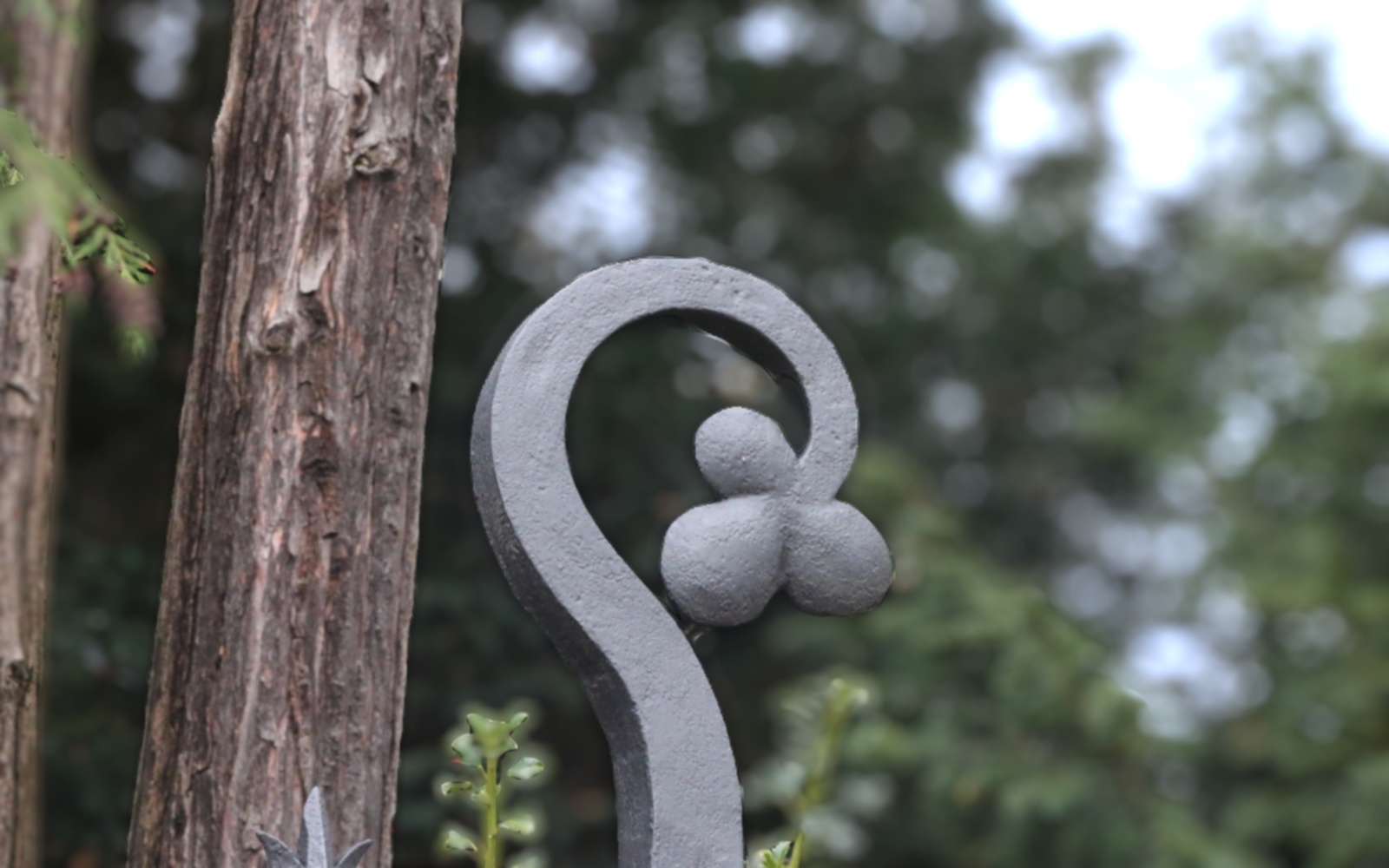
Select camera
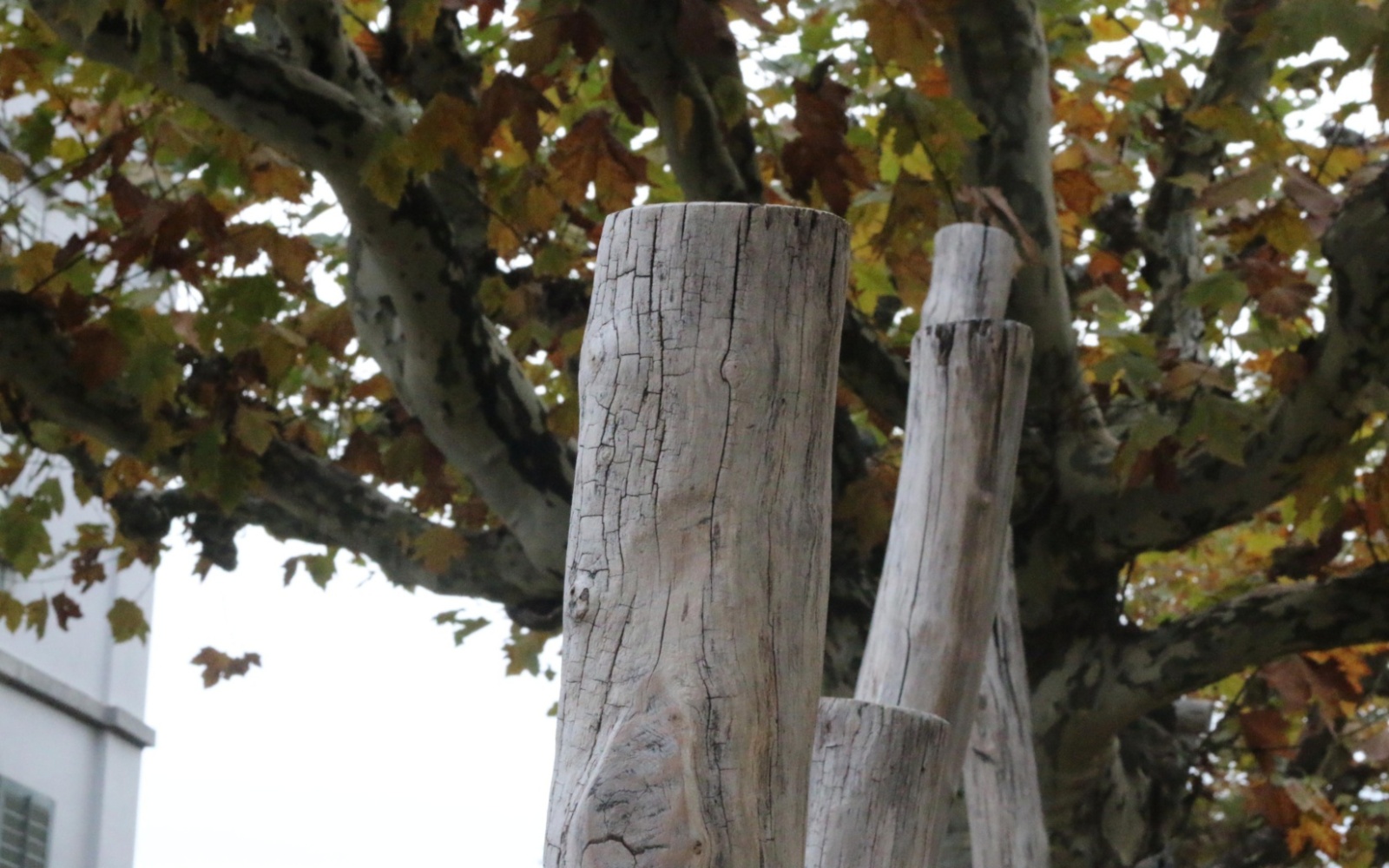
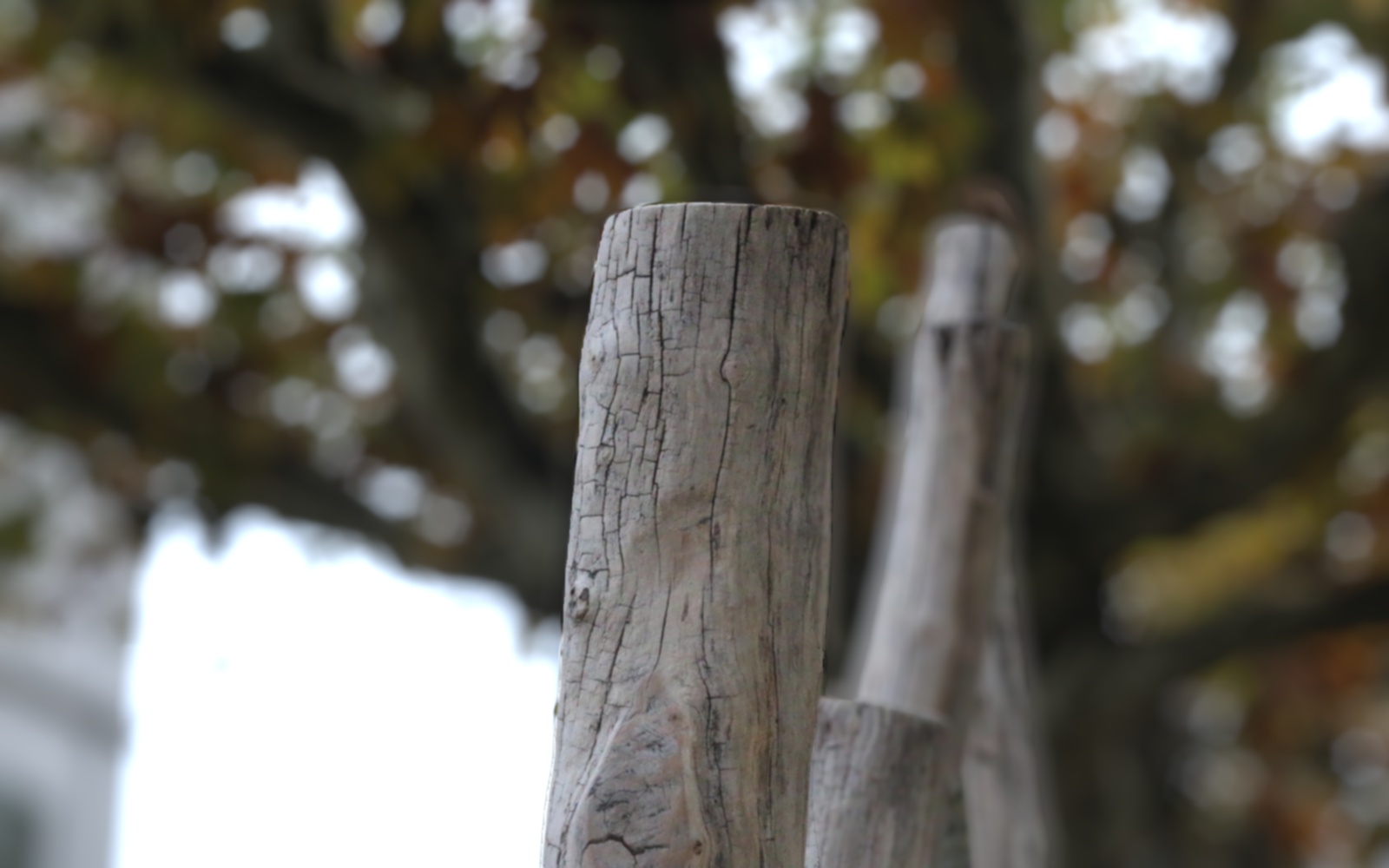
Select camera
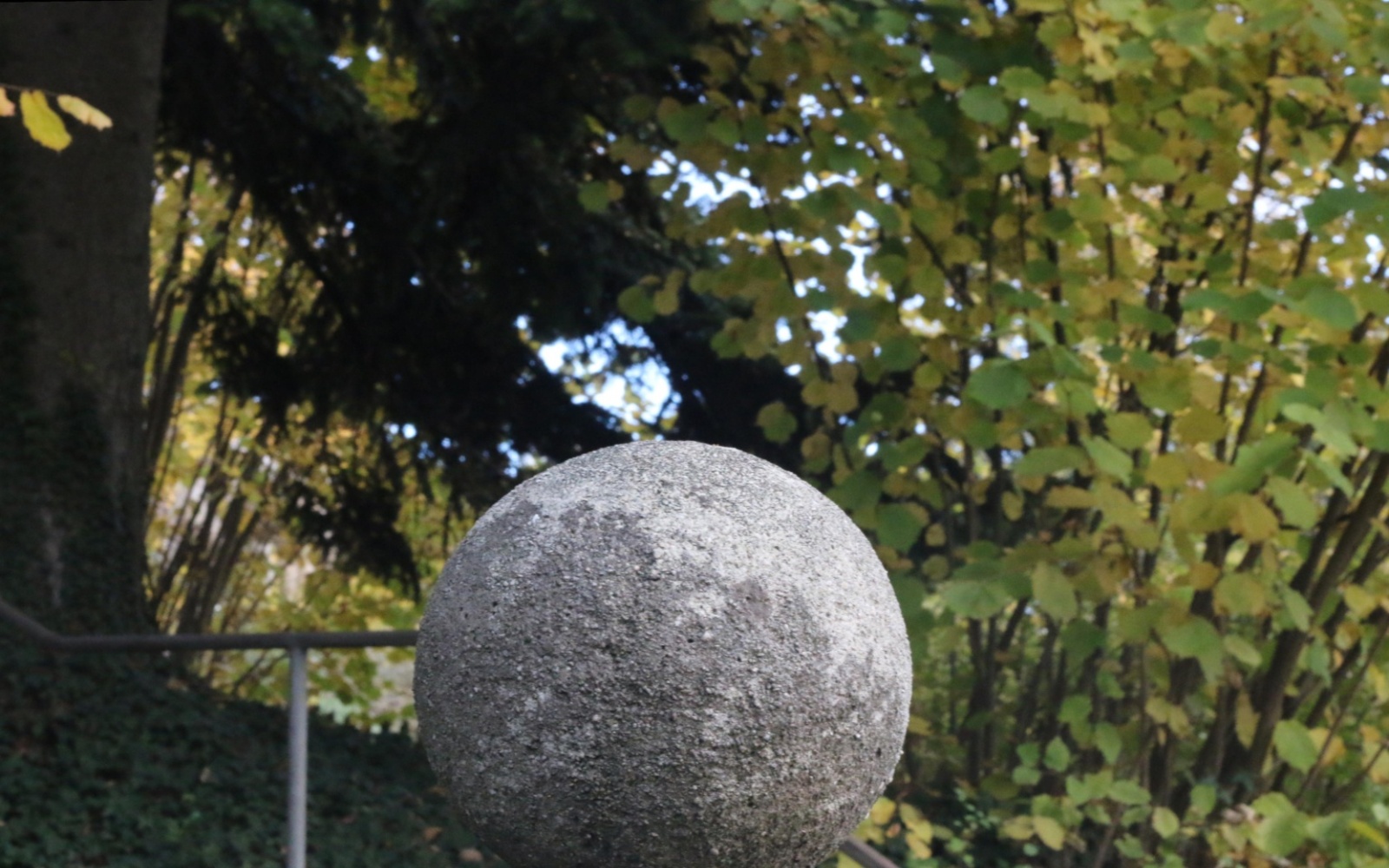
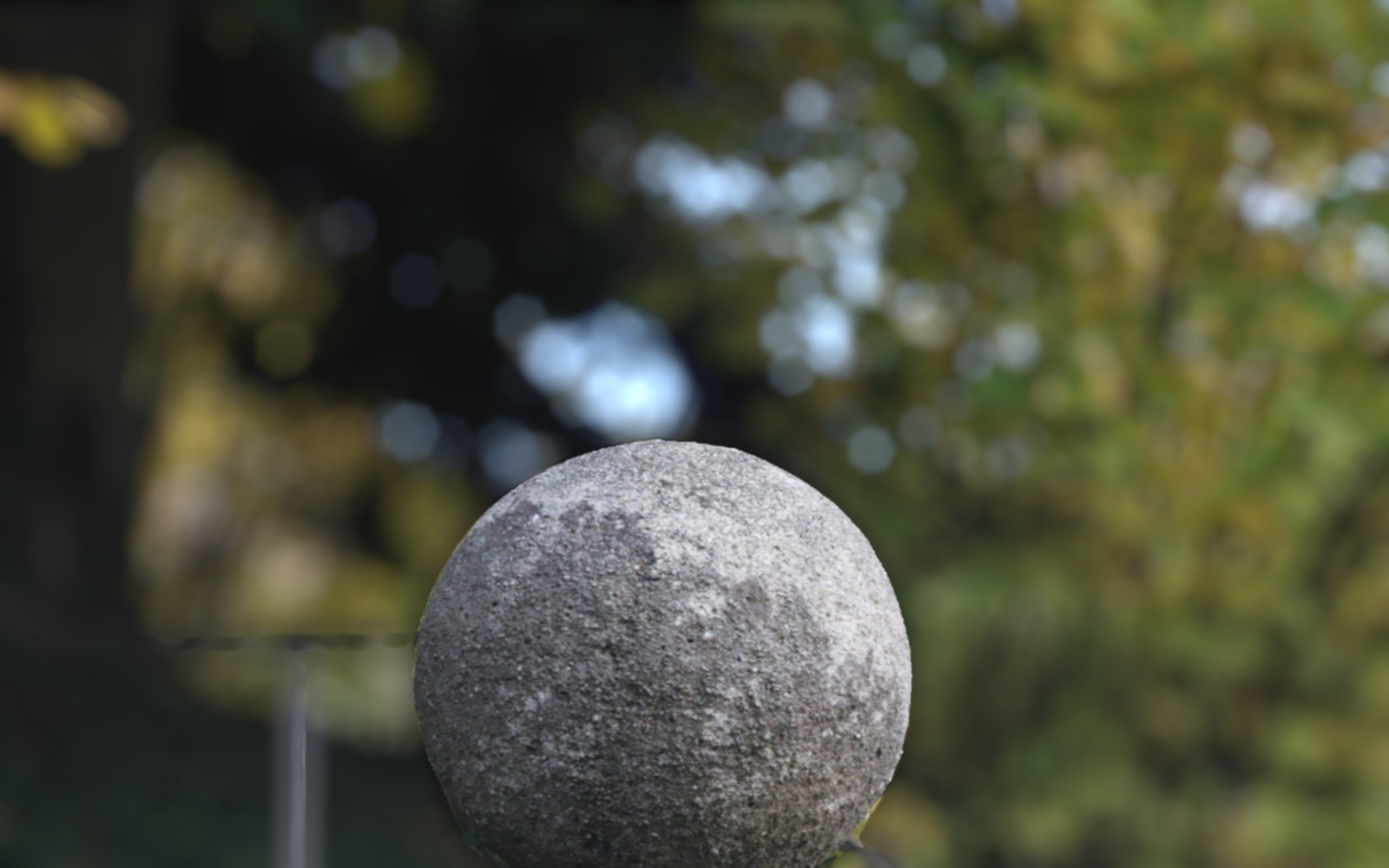
Select camera
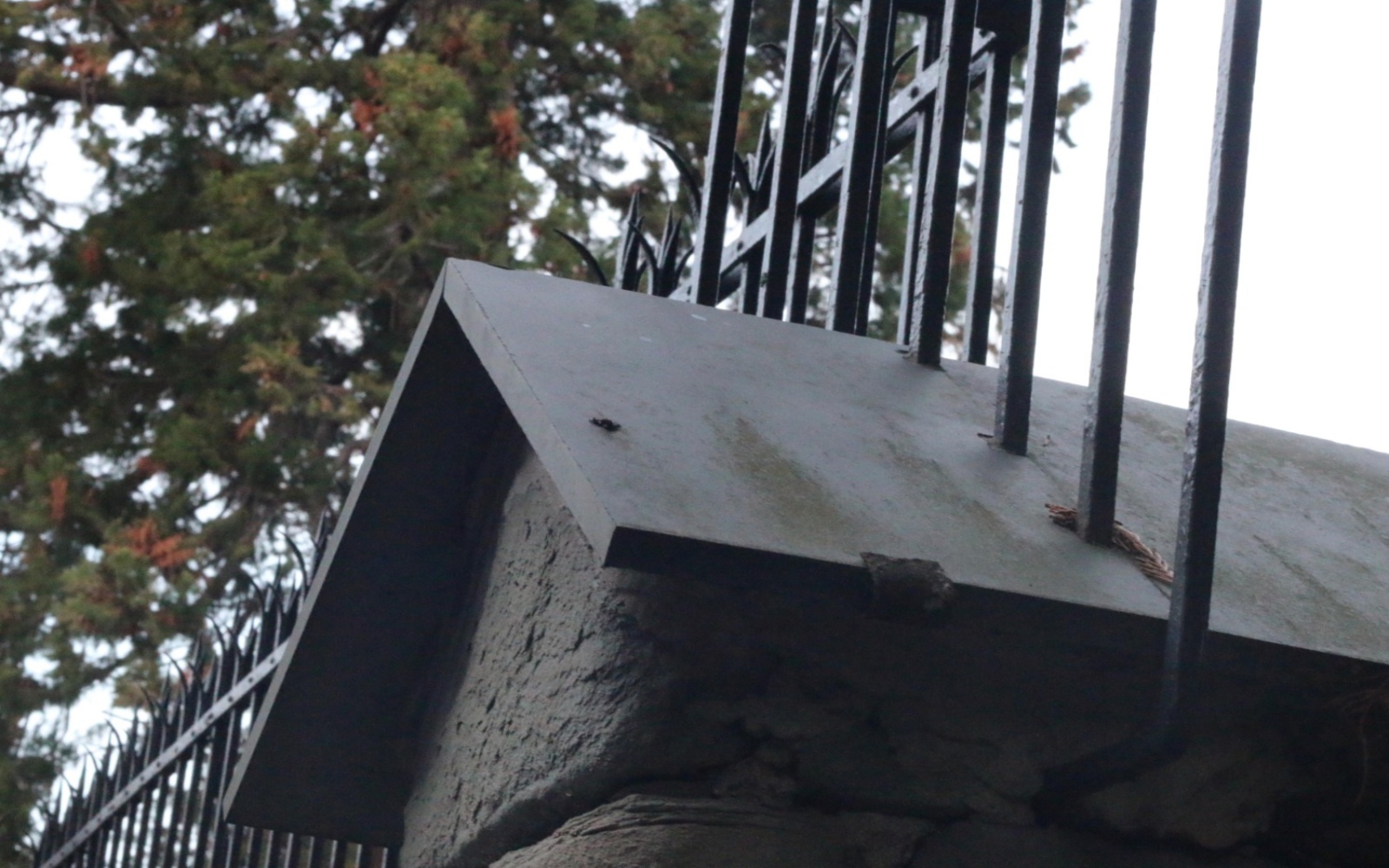
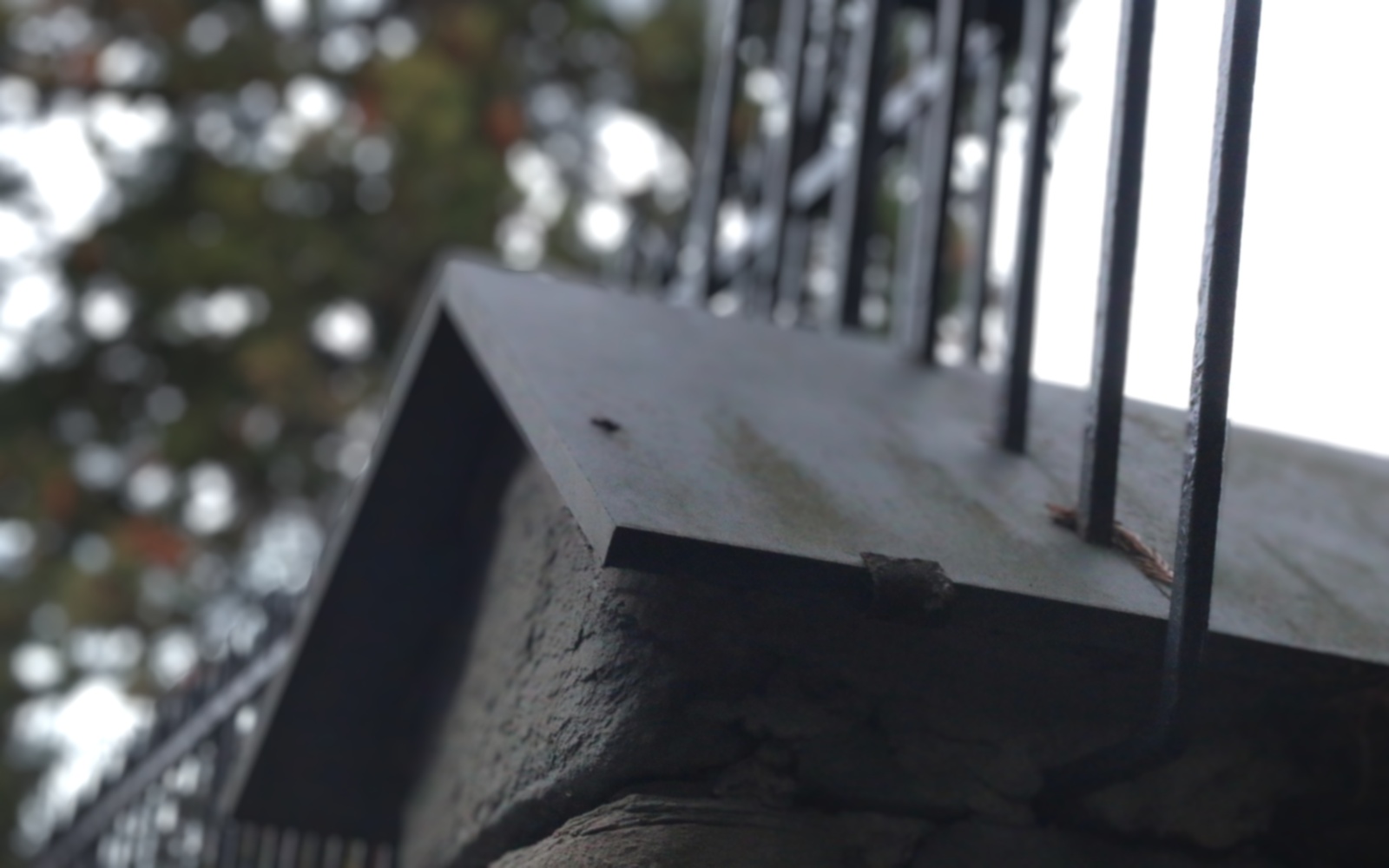
These examples show the output of our system when applied to all-in-focus images. The depth here is computed using DPT. Use the box to select which camera model should be applied.
Refocusing
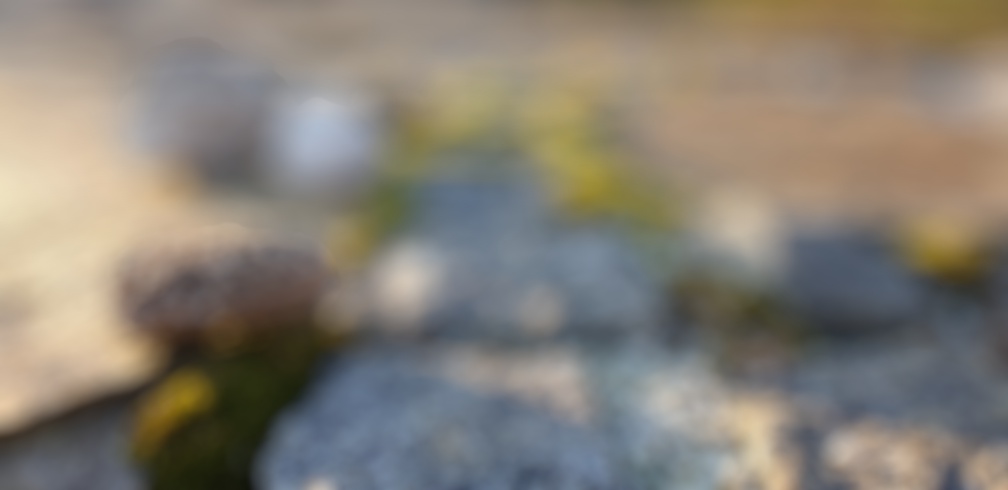
Select camera
Focus distance
This examples shows a focus sweep applied to an all-in-focus image. The depth of field is computed using the selected camera model.
Compositing
This example shows a rendered carousel that is blurred using our system. The result is that added to an image of the camera that our system has been trained for. Notice how the animated highlights of the carousel change depending on the position in the image.
Related Projects
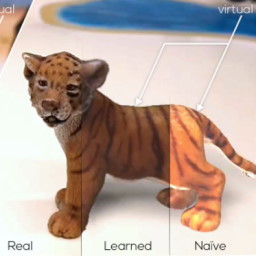
Neural Cameras: Learning Camera Characteristics for Coherent Mixed Reality Rendering
Coherent rendering is important for generating plausible Mixed Reality presentations of virtual objects within a user’s real-world environment. While existing approaches either focus on a specific camera or a specific component of the imaging system, we introduce Neural Cameras, the first approach that jointly simulates all major components of an arbitrary modern camera using neural networks.
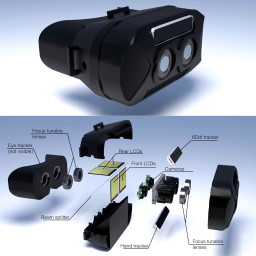
Video See-Through Mixed Reality with Focus Cues
This work introduces the first approach to video see-through mixed reality with full support for focus cues. By combining the flexibility to adjust the focal distance found in varifocal designs with the robustness to eye-tracking error found in multifocal designs, our novel display architecture reliably delivers focus cues over a large workspace.
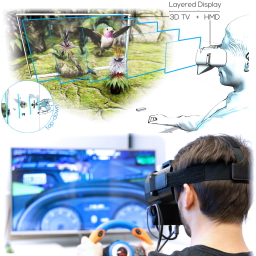
Off-Axis Layered Displays: Hybrid Direct-View/Near-Eye Mixed Reality with Focus Cues
This work introduces off-axis layered displays, the first approach to stereoscopic direct-view displays with support for focus cues. Off-axis layered displays combine a head-mounted display with a traditional direct-view display for encoding a focal stack and thus, for providing focus cues.
Citation
@inproceedings{Mandl2024NeuralBokeh,
author={Mandl, David and Mori, Shohei and Mohr, Peter and Peng, Yifan and Langlotz, Tobias and Schmalstieg, Dieter and Kalkofen, Denis},
booktitle={2024 IEEE Conference on Virtual Reality and 3D User Interfaces (VR)},
title={Neural Bokeh: Learning Lens Blur for Computational Videography and Out-of-Focus Mixed Reality},
year={2024},
}